报告时间:3月22日(星期五)9:00
报告地点:八角楼学术报告厅
(一)
报 告 人:Witold Pedrycz,IEEE Fellow,加拿大阿尔伯塔大学教授

(Prof. Wtiold Pedrycz, University of Alberta, Canada)
报告题目:Generative and Discriminative Facets of Granular Data
内容简介:Concepts constitute a concise manifestation of key features of data. As being built at the higher level of abstraction than the data themselves, they capture the essence of the data and usually emerge in the form of information granules. Data descriptors-information granules characterize data in a concise way and are further used as functional building blocks (modules) of classifiers or predictors.
Generative and discriminative aspects of information granules and information granularity deserve detailed investigations. Generative aspects of information granule are concerned about a representation of data by a collection of information granules. Discriminative aspects of granules deal with their abilities to realize mappings of classification (discrete) or predictive (continuous) character.
The systematic process of design of information granules in the presence of numeric data is elaborated on. Two phases are delineated: first a collection of numeric representative (prototypes) is formed with the aid of clustering algorithms. In the sequel, they are augmented to information granules with the help of the principle of justifiable granularity. Several variants of this principle are discussed to reflect the essence of the underlying problem and some requirements of the problem.
The discriminatory nature of information granules is illustrated in the general setting of granular classifiers and the problem of transfer learning.
Furthermore we elaborate on the general paradigm of granular modeling dwelling upon a collection of information granules and discuss a phenomenon of a successive elevation of type of information granularity emerging through refinements of numeric and granular models.
报告人简介:Witold Pedrycz (IEEE Fellow, 1998) is Professor and Canada Research Chair (CRC) in Computational Intelligence in the Department of Electrical and Computer Engineering, University of Alberta, Edmonton, Canada. He is also with the Systems Research Institute of the Polish Academy of Sciences, Warsaw, Poland. Dr. Pedrycz is a foreign member of the Polish Academy of Sciences and a Fellow of the Royal Society of Canada. Witold Pedrycz has been a member of numerous program committees of IEEE conferences in the area of fuzzy sets and neurocomputing. He is a recipient of the prestigious Norbert Wiener award from the IEEE Systems, Man, and Cybernetics Society, IEEE Canada Computer Engineering Medal, Cajastur Prize for Soft Computing from the European Centre for Soft Computing, Killam Prize, and a Fuzzy Pioneer Award from the IEEE Computational Intelligence Society.
His main research directions involve Computational Intelligence, fuzzy modeling and Granular Computing, knowledge discovery and data mining, fuzzy control, pattern recognition, knowledge-based neural networks, relational computing, and Software Engineering. He has published numerous papers in this area. He is also an author of 16 research monographs covering various aspects of Computational Intelligence, data mining, and Software Engineering.
Dr. Pedrycz is vigorously involved in editorial activities. He is an Editor-in-Chief of Information Sciences, Editor-in-Chief of WIREs Data Mining and Knowledge Discovery (Wiley), and Int. J. of Granular Computing (Springer). He serves on an Advisory Board of IEEE Transactions on Fuzzy Systems and is a member of a number of editorial boards of other international journals.
(二)
报 告 人:陈俊龙,IEEE Fellow,澳门大学教授
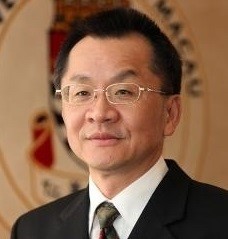
(Prof. C. L. Philip Chen, University of Macau, China)
报告题目:Structural Variations in Broad Learning System and Regularized Robust Modelling
内容简介:After a very fast and efficient discriminative Broad Learning System (BLS) that takes advantage of flatted structure and incremental learning has been developed, this talk will discuss the framework of several BLS variants with their mathematical modellings are given. The variations include cascade, recurrent, and broad-deep combination that cover existing deep-wide/broad-wide structures. In addition, regularized robust modeling in BLS will be discussed. From the experimental results, the BLS and its variations outperforms several exist learning algorithms on regression performance over function approximation, time series prediction, face recognition, and data modelling.
报告人简介:陈俊龙(C. L. Philip Chen)博士,我国自动化学会副理事长,国家特聘专家,澳门科协副会长,澳门大学讲座教授,科技学院前院长。陈教授是IEEE Fellow(院/会士)、美国科学促进会AAAS Fellow(院/会士)、国际模式识别 IAPR Fellow (院/会士)、欧洲科学院院士、欧洲科学与艺术院院士、国际系统及控制论科学院 IASCYS 院士、自动化学会(CAA)、及香港工程师学会 (HKIE) Fellow。陈教授现任IEEE系统人机及智能学会的SCI期刊主编(IEEE Trans. on Systems, Man, and Cybernetics: Systems),曾任该学会国际总主席 (2012-2013)。陈教授主要科研在智能系统与控制,计算智能,混合智能,数据科学方向。在2018年“计算机科学学科”高被引用文章数目学者中全世界排名在前 17名。也是Clarivate Analytics的全球高被引科学家。详见 https://orcid.org/0000-0001-5451-7230。陈教授的科研包括国家基金委重点项目及面上项目在人工智能方向的科研,以及多项澳门科学基金委的资助,并获得两次澳门自然科学奖。陈教授是美国工学技术教育认证会(ABET)的评审委员。 澳门大学工程学科及计算机工程获得国际【华盛顿协议】及【首尔协议】的认证是陈教授对澳门工程教育的至高贡献。担任院长期间带领澳门大学的工程学科及计算机学科双双进入世界大学学科排名前200名。2016年他获得了母校,美国普度大学,的杰出电机及计算机工程奖。2018年他获得了IEEE系统科学控制论的最高学术维纳奖 (Norbert Wiener Award)。